Anyhow one of the first tasks I have been asked to look at is monitoring the server. Now Tableau comes with some dashboards to look at things like user activity, disk space and extracts. These do a pretty good job of saying what's currently going on, but you soon find out that you need a bit more information and that's the point of these posts, to walk through what I needed and how I did it.
First up, Extracts
We use lots of extracts on our server for a number of reasons. A lot of our data is not from fast warehouses, in a lot of cases, it's from the live databases so we need a way to get the data and make sure that the user interaction in the dashboards is as fast as possible, so that's why we extract. When looking at the extracts I have got 3 tasks that I want to do
- Identify and fix all failing extracts, find those one-off errors and put measures in place to stop them happening again. Fix those that are constantly failing due to connection passwords changing etc.
- Once all the failures have been addressed the next step is to look at usage, how many of these extracts are being used, what's the total number of monthly views , what is the size. Those old extracts can be turned off to reduce load and space on the server and remove duplicates
- Now we just have the extracts that are needed, they don't fail so the final step is to optimise them, reduce the complexity, increase performance, and tune them up.
- Use the new v10 alerts function to tell users when their extracts have failed so that they can monitor it themselves. Self service FTW!
So the first little issue is over failing extracts. Tableau server comes with a nice little dashboard that shows "How Have Extracts Performed on This Sever" and it lets you select some filters and find extracts that have fallen over or that have taken a long time. The first job I have been asked to do is find out why these are failing and either fix them or at least know what's going on.
So what I can see is that we have roughly 100 failures every day,now is that good or bad? Are that 100 extracts failing once, or one extract failing 100 times. Are they old or new, are they even being used any more? Like most things related to Tableau, one answer always leads to more questions. There is a separate tab to look at the amount of traffic to a data source, but then that wouldn't list the ones that have failed. As this is a pretty specific query it looks like I'll have to build my own dashboard to assist. And that's never a bad thing, always nice to roll up the sleeves and build something.
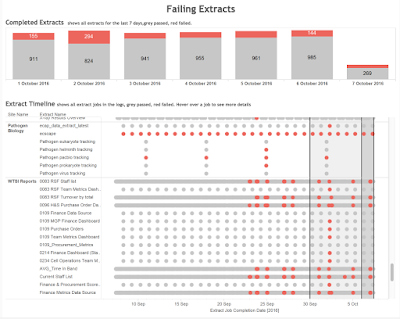
But crucially, we need to know whether the failing extract was a one-off or if it's always failed or something that has recently fallen over. It's this that's the important thing to look at with any error. Problems like disk space, they might come and go as old data gets pruned but changes to database names might be reoccurring issue. Just getting a list of the current fails doesn't give enough information. I've added the grey bars using reference lines to show today in dark grey and the last 7 days in the lighter grey so that it tied it back to the overview bar charts at the top. This also helps to quickly see if the issues are recent of have been going on for days or weeks.
Then, of course, the next step is, so this extract is failing, but what is the error, who published it, when did it last correctly run and is it even being used anymore. All this comes from the postgresDB and added to the tooltip
So now I have a single dashboard that in a glance I can see if we have site-wide issues, local project issues, a re-occurring issue that needs intervention, or just a blip that will sort itself out.
Once I have all the monitoring dashboards in place to check that things are not failing, I can then go on to the next step and look at usage, remove those that are not needed anymore, and then finally tune those that are.
If these next steps go as well as the first, then I will have made a huge step in getting the server under my command. Muhahahah